Data-informed decision-making means getting timely intelligence for the task at hand. For instance, if you’re running a business, the kind of timely intelligence you’re looking for could be:
- “Which of my prospect accounts are engaging well with my marketing campaigns and which aren't?”
- “Which of the 100 prospect accounts in my territory do I have a better chance of closing with this quarter?”
- “Should I be reaching out to Company A today or Company B?”
Today, modern marketing and sales teams approach answering those questions in a variety of ways. Unfortunately, those methods put a lot of responsibility on the person with the question to dig into the data. It can overwhelm reps, teams, and departments to include more first- and third-party data sources, implement new tools, create dedicated data-centric roles, and transform their data pipeline.
Oftentimes, these teams might be working with operations to get these answers, but with backlogs and competing requests, those teams are also overrun with work. Supporting marketing and sales teams to know what to do today might not be top priority.
Instead, teams piece together data from different sources or pouring over dashboards to create the best view they can of what's happening. Yet, anyone who’s been frustrated spending hours sifting through Netflix titles, looking for something to watch and then finally giving up without making a choice, can empathize with the information paralysis that many teams feel.
It's also important to consider how the human brain processes information to make decisions. Instead of overwhelming our brains with lots of visual stimuli, teams need to filter out extra context or unrelated data to focus on information in pieces that can be easily understood and processed with their goals in mind.
Marketing and sales reps can be better supported with meaningful and useful abstractions. In this case, metrics can summarize or aggregate raw data for a specific task. Well-designed metrics can ease the process of digesting information quickly.
Let’s walk through creating a few examples of metrics and examine their utility.
Creating Useful Metrics
Consider the first question from the first paragraph: “Which of my prospect accounts are engaging well with my marketing campaigns and which aren’t?”
Option 1
To answer this question, let's start with a potential simple metric called campaign_responses_last_1_year. This metric could store the computed sum of the total responses for all campaigns for a year for an account. You could then sort accounts in descending order by this metric’s value. The top N accounts could then be your best performing accounts and the bottom N could be your worst accounts.
However, this simple first pass is not a very sophisticated take. A one year aggregate doesn’t tell you how accounts are currently engaging or have engaged recently. At the same time only considering the most recent data (e.g., the last 7 days) may be too susceptible to a recent spike or period of inactivity.
Option 2
To balance the two considerations, metrics based on moving averages could do a better job, as they tend to smooth out spiky data. Some examples include metrics such as
- campaign_responses_rolling_14_day_average: This example encodes the moving 14-day rolling average for campaign responses.
- campaign_responses_rolling_4_week_average: This metric encodes the weekly average.
You could define daily (e.g., 14-day average), weekly (e.g., 4-week average), or weekly rolling averages to summarize recent campaign performance.
Option 3
A metric with a higher degree of sophistication could consider all accounts not being created equally. For instance, some accounts might represent companies with 1000s of employees and others might have only 10 employees. Normalizing the campaign responses with the number of contact records per account might be another way to compare performance across accounts. We could call this metric campaign_responses_per_contact_rolling_average.
Once you’ve computed the above metric for all of your prospect accounts, you use it to sort your list of accounts. Top accounts are candidates for targeted engagement since the metric could likely be signaling a greater degree of interest. Accounts at the bottom might require a change in strategy. That could mean pausing campaign activity or creating new campaigns for better engagement.
Looking forward
With well-defined metrics, marketing and sales teams can get the information they need to get work done more effectively and efficiently.
In later posts, we will expand on designing metrics, enabling and deploying them at scale, and ways on making them actionable for organizations of all sizes.
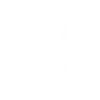