When you hear the word “prediction,” it’s easy for your mind to be filled with images from the future swirling in a crystal ball. Someone gazed into the future and figured out what was going to happen, or maybe they were so bold as to try and see how their own actions could lead to different outcomes. As compelling as this image can be, humans are limited, or perhaps blessed, to be locked in the present, unaware of what’s to come — and predictions are no different.
Whether it’s a human making a prediction, or a machine learning model, the process is generally the same. The entity will look at things that have happened in the past, tie those events to a particular outcome, and assume that when the relevant events do or don’t happen, the outcome will be more likely.
If the sky gets dark and cloudy, it will probably rain.
If a basketball team is up by 20 points at half, they’ll probably win.
If someone has gotten lunch from McDonald's every Friday for the past 10 weeks, they will probably do the same this Friday, too.
But sometimes it gets cloudy and doesn’t rain, sometimes a team will blow a large lead, and sometimes people get tired of their usual restaurants. This doesn’t make the predictions bad, it just underscores their real purpose.
Predictions are tools, tools that can be very powerful when properly used. If it’s cloudy, it’s a good idea to bring an umbrella. It might not rain, but it’s better to have an umbrella and not need it than to be unprepared. The prediction allows you to make a decision you wouldn’t have otherwise, a decision that has the potential of a large benefit at little-to-no cost.
Other situations have risks and costs that aren’t quite as simple. If the basketball team is up by 20 points at half, it might not be a bad idea to bet a modest amount on them winning, but it would still be irresponsible to bet your life savings. Each prediction will have its own unique purposes, risks, and rewards.
At Klearly, we make predictions to help revenue teams know who to target next. These insights are powerful suggestions meant to augment a professional’s experience, expertise, and judgment, but not replace it.
A predictive model is able to analyze the data it sees and learn complex patterns that would be impossible for a human to detect at scale. A human is able to analyze some data points the model may never see, such as the tone in someone’s voice, what was discussed on a call, and what current events are happening that might impact the future.
If the model says a customer is unlikely to buy, but they show significant interest on a phone call, it’s okay to set the prediction aside and trust your judgment, we promise we won’t be offended.
But just as often, there will be times when you won’t know who to reach out to. Or the thousands of unengaged accounts in your system are too much for a human to sort through. Or you’ll have dozens of engaged accounts you have to juggle. These are the moments where Klearly predictions are especially useful.
No one will ever be able to tell you with certainty who will buy what and exactly when that purchase will be made, but our predictions are like having an umbrella handy on an overcast day — it's better to know what's likely to happen so you can be prepared for that situation.
Want to learn more about how we think about predictive models at Klearly? Check out this interview with Michael as he explains using data to predict retention and churn.
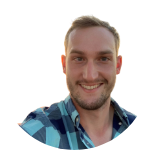