While many revenue leaders rely on their gut or industry benchmarks to forecast expected retention, what if we could have extra insight into when we’d need to apply extra effort to retain our current customers? With so much data available, knowing how we can activate that information can be a helpful tool about how to make the biggest impact.
To find out what we can learn from our data, I sat down with Klearly’s Data Scientist Michael Dillon who talked through the basics of how to predict when a customer might drop a product and how revenue teams can work with predictions to get an edge.
Sam: Is it possible to predict when a company might lose a customer?
Michael: As with most questions worth asking, it depends, but yes, data can help predict retention and churn. With the right data and enough of it, a model can make good predictions that can help revenue teams make better decisions on how and where to prioritize their time and efforts. There will be limitations to those predictions no matter what, but understanding those limits could help know when it’s time to act.
What expectations should someone have about the accuracy of modeling predictions?
One of the most important things to understand about predictive modeling is that we’re talking about something that hasn’t happened yet. Models have gotten fairly good at simple tasks like identifying a cat or a dog in a picture because that’s clearly right or wrong. What we’re doing doesn’t have a correct answer because the future hasn’t happened yet. Just like a meteorologist isn’t always right, the information they give us about the weather can be helpful at a low cost to us, for example picking up an umbrella before we leave our house in case it might rain. Models like ours won’t ever be 100% accurate but can do a fairly good job of finding nuanced patterns depending on the data you have.
What kind of data is important when thinking about optimizing your revenue team for retention?
Best case scenario is when a data set is rich and there’s a lot of it. For example, a B2B software company knows their customers’ industry, company size, financial data, and even product usage data including how often users are engaging with the product and what features they are using most.
If you have all of that information, you know who the customer is, how they’re doing, and how heavily they’re using the product. In that case, you could be relatively confident that predictions will be good, even though there will always be outliers. Some customers who use your product and are doing well will leave for another reason that you can’t anticipate or other customers who rarely engage with your product but stay for the value of a question answered.
At Klearly, we typically begin by looking at the interactions between current accounts and how they're engaging with sales, marketing, and customer support teams. When we can see what they're engaging with from an inbound and outbound perspective, it tells part of the story. Where it gets really exciting is being able to add in other available data sources that can help the model find patterns. As mentioned, that might include firmographic information, product telemetry data, and more.
In the place of models telling revenue teams what to prioritize, many have created indicators like tiers or flags. Can you talk about your thoughts about those types of data points?
As humans, we’re very, very good at finding patterns, so there are likely lots of measures used regularly by professionals with domain knowledge that are useful in their own right and can be used to make the model better. Whether teams are using a custom loyalty measurement or something more common like a NPS (Net Promoter Score), more data provides an opportunity for the model to find the nuance or minor patterns we’d otherwise miss.
One of the most common issues I see for businesses in any domain is to create simple models or rules where intuition isn’t ever checked against real data or compared against the outcome that actually matters. There can be some really logical rules that just don’t pan out in the data.
For example, marketing might have a rule that designates an account as “Marketing-Qualified” based on a number of engaged contacts from that account. At first glance, this rule makes a lot of sense. However, how many engaged contacts is the “right” number? Five engaged contacts might be the right threshold for a large company with many people involved in the buying process, but it may be too many for a small company with a more compact buying group.
Rules like this one are helpful in some cases, but don’t have a good way of assessing the precise needs based on all the other account information available. When teams continue to rely on rules or intuition unchecked they might lead themselves into pursuing arbitrary targets that aren’t tied to the outcomes they’re looking for.
Are there any other issues you see when it comes to business leaders understanding model predictions?
Yes, there’s a lot of psychology around the perception of predictions. If you are focused on a percentage, for example, if you think of an 85% chance of retaining a customer as a sure bet, there’s still a good possibility that it won’t happen somewhat often. At Klearly, we don’t use percentages when showing predictions to prevent that confusion.
In the end, predictions can’t replace the expertise of professionals. No person or model is able to predict the future. But working together, models—especially when paired with helpful messages—can provide teams with information they wouldn’t normally have to help them decide where they can get the biggest impact from their time and efforts.
Want to learn more about how our customers are using data to receive helpful insights they can use? Request a demo →
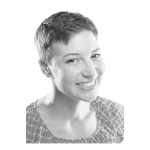